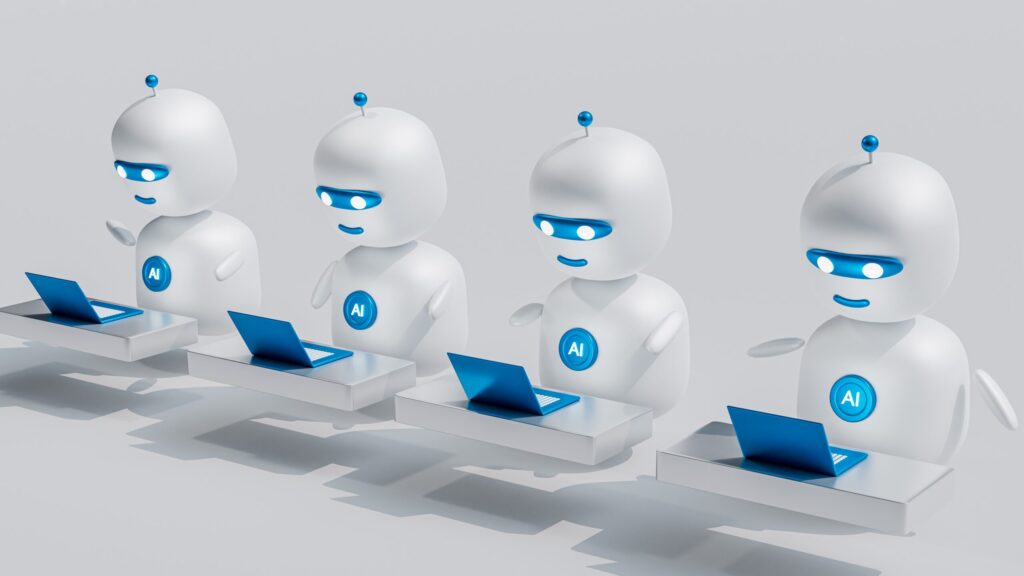
ChatGPT: stochastic parrot or helpful tool?
04.07.2023
Despite all the amazement about ChatGPT, we should not forget that the software is based purely on pattern processing and generates linguistic utterances without any reference to world knowledge. Basically, the model calculates transition probabilities for sequences of words, explains Prof. Ute Schmid, Head of Cognitive Systems Group at the University of Bamberg. In our interview, the psychologist and computer scientist describes how ChatGPT works and what limitations the bot has. In her view, the use of AI systems makes sense when they advance society, for example, to improve people’s quality of life or enhance their competences.
We are currently experiencing the umpteenth fuss about artificial intelligence. What could be the reason for this?
I think that the low-threshold possibility of interacting with ChatGPT has made it possible for a broad audience to experience the power of an AI technology for the first time. The same is true for image generators like DALL-E. The previous hype, which was mainly triggered by the impressive successes in the classification of images, only reached the general public indirectly, via media reports. Thus, the discussions about AI in image-based medical diagnostics – for example, for skin cancer detection – or pedestrian detection remained rather abstract.
What do you understand by artificial intelligence?
One textbook definition is: „AI researches how to enable computers to do things that humans are currently better at solving.“ The motivation that led to the founding of the research field in 1956 by computer pioneer John McCarthy was the assumption that many aspects of human intelligence can be formalised by algorithms and simulated accordingly as computer programs.
AI methods are used when it is not possible to apply standard algorithms.
On the one hand, this is the case with problems where it is not possible to compute their solution efficiently – i.e. in a reasonable amount of time. In this case, so-called heuristic methods – one of the basic family of methods in AI – are used to compute approximate solutions.
And on the other hand?
On the other hand, AI methods, especially machine learning, are needed when it is not possible to describe the problem exactly. This is the case, for example, for the recognition of objects in images. It is incredibly easy for us humans to recognise that the object in front of me is a cat, or for medical professionals this mole could be skin cancer. We humans use such knowledge implicitly, we cannot describe it fully in language. For this task models are learned, for example with neural networks, which are then used instead of explicit programs and determine for an image what kind of object can be recognised on it.
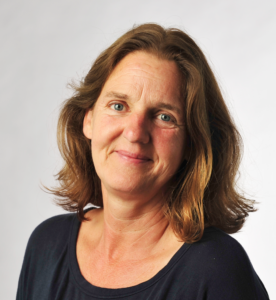
Photo: Jürgen Schabel
In which subarea do you classify ChatGPT and how does this software work?
ChatGPT belongs to the family of generative models. This is a special class of neural networks, so-called transformers. The basis is the large language model GPT3, generative pretrained transformer, which was trained on hundreds of billions of words with so-called self-supervised learning. All content accessible on the internet – from Wikipedia to blog posts – up to 2021 was used. The core of the model is that it computes transition probabilities for sequences of words. This gives it considerable ability to formulate sentences in different languages. Based on GPT3, the dialogue system was then built. This uses different types of machine learning. For example, content that involves violence, racism or sexism is filtered, and the filters were trained with supervised learning.
Specifically, click-workers from Kenya in particular have had to mark what is toxic content in countless texts in order to train the filter.
When we use ChatGPT and give feedback on how good the response was – via the thumbs-up icon – this information is used for refinement. This method is called human-in-the-loop reinforcement learning. You can also see that for numerous queries for which incorrect answers were given two months ago, correct answers are now provided.
As it stands, ChatGPT would pass The Turing Test without a hitch and thus prove that a computer can no longer be distinguished from a human being or his ability to think. Your colleague Prof. Judith Simon, member of the German Ethics Council, recently criticised that this confuses language performance with thinking performance. How do you see that?
I absolutely agree with that. ChatGPT is based purely on pattern processing, it does not „understand“ our questions and sees no difference between a question about a love poem or a physical fact.
We humans tend to be bluffed by systems that are agent-like.
We have a tendency to anthropomophize systems and ascribe to them mental states similar to our own.
So why should we not expect ChatGPT to produce texts with a reference to truth?
In principle, linguistic utterances are generated without reference to world knowledge or domain-specific knowledge, such as medicine or mathematics. For example, if one enters the information that Anna and Max are going for a walk together and that Max sees a cat and Anna pets the cat, ChatGPT answers the question of who saw a cat with Max.
Human readers conclude from the fact that Anna is petting the cat that she has also seen it.
It should also be noted that content from the Internet has been incorporated into the language model GPT3 without being checked. For some topics, there are probably serious texts on the net. For other areas, on the other hand, it may well be that the amount of dubious content predominates. If you compare ChatGPT with a search engine, ChatGPT does not provide the context in which the information appears. For example, if I use Google to search for remedies for insomnia, I might be offered the page of a university clinic that conducts sleep research or the page of a pharmaceutical company. Most of us have enough media literacy to weigh up how much we trust what content. This context is missing from ChatGPT. The content from countless websites has been transferred into a huge language model from which no reference back to the original source is possible.
The new deep learning approaches are data-intensive because many training examples are needed for learning. Why is the conclusion not correct: a lot of data results in correct or fair models?
Correctness cannot be assumed for models built with machine learning, since they are generated by generalisation over a sample of data. However, with regard to GPT3, it has been shown that the size does have a positive influence on the quality. If you use all the content there can possibly be used to build a model, then you have created a stochastic parrot. In general, the quality and distribution of the training data has a major impact on the quality of a learned model. For example, if they learn from historical data which application profiles led to hiring for certain jobs, women may not be invited when applying for software development jobs. This was the case with Amazon’s recruitment tool in 2015. The sampling biases in training data, as in Amazon’s case, can be reduced with careful data sampling, but one can never completely rule out such unfair biases.
It should also be noted that content from the Internet has been incorporated into the language model GPT3 without being checked. For some topics, there are probably serious texts on the net. For other areas, on the other hand, it may well be that the amount of dubious content predominates. If you compare ChatGPT with a search engine, ChatGPT does not provide the context in which the information appears. For example, if I use Google to search for remedies for insomnia, I might be offered the page of a university clinic that conducts sleep research or the page of a pharmaceutical company. Most of us have enough media literacy to weigh up how much we trust what content. This context is missing from ChatGPT. The content from countless websites has been transferred into a huge language model from which no reference back to the original source is possible.
In which fields can AI systems be helpful, and in which will humans be indispensable?
I would like to start here with an example: In a project funded by the German Federal Ministry of Education and Research we looked at the topic of mobility in old age. One could consider using AI methods to help people with limited mobility to do their shopping, analyse what is still in the fridge, place the order and have it delivered. But that would have the unpleasant side effect that the elderly person, who may live alone, would become lonely. Instead, in the project, we developed a match-making service based on AI methods, which brings older or impaired people together with people who would like to volunteer. In my opinion, a shopping companion makes a greater contribution to the quality of life than having groceries delivered.
AI methods can be used in different ways in virtually every area of life. Whether this is good for people depends on the socio-technical embedding. What is needed here is a broad democratic discourse with those affected, professional associations and AI researchers in order to sound out which AI systems contribute to the well-being or competence enhancement of people and which do not.
In general, I am against empathy simulated with AI methods. Anyone who uses ChatGPT to write a love letter or a condolence letter is, in my opinion, socially depraved. Using ChatGPT to write a customer information letter, on the other hand, is efficient. One of the best effects we have seen with the introduction of ChatGPT, in my opinion, is that we are finally discussing the issue of education. We should all learn to use these technologies wisely and at the same time design education to promote judgement, problem solving skills and knowledge transfer.
Neueste Beiträge
- Frankfurt fährt vorne: Deutschlands fahrradfreundlichste Stadt 23. Juni 2025
- Pestizide: EU verlängert Zulassung ohne Risikoprüfung 16. Juni 2025
- Stadtplanung: Verkehrswende bringt Kaufkraft 23. Mai 2025
- Smartes Materialdesign gegen Plastikflut 15. April 2025
- Bionische Roboter: fliegen wie Schmetterlinge 3. März 2025
- Stromspeicher aus Beton 7. Februar 2025
- Null Energiebedarf: adaptive, bionische Gebäudefassaden 29. Januar 2025
- Apps: persönliche Informationen landen bei Datenhändlern 16. Januar 2025
- Leben in leerstehenden Warenhäusern 13. Januar 2025
- Energiewende: bezahlbare Alternativen statt Bargeld 30. Dezember 2024